Machine Learning for OpenCV by Michael Beyeler
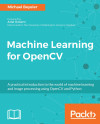
Privacy Policy
Read using
(price excluding SST)
Title:
Machine Learning for OpenCV
Author:
Michael Beyeler
Category:
Engineering & IT
ISBN:
9781783980291
Publisher:
Packt Publishing
File Size:
26.46 MB
(price excluding SST)
Synopsis
Key FeaturesLoad, store, edit, and visualize data using OpenCV and PythonGrasp the fundamental concepts of classification, regression, and clusteringUnderstand, perform, and experiment with machine learning techniques using this easy-to-follow guideEvaluate, compare, and choose the right algorithm for any taskBook DescriptionMachine learning is no longer just a buzzword, it is all around us: from protecting your email, to automatically tagging friends in pictures, to predicting what movies you like. Computer vision is one of todays most exciting application fields of machine learning, with Deep Learning driving innovative systems such as self-driving cars and Googles DeepMind.OpenCV lies at the intersection of these topics, providing a comprehensive open-source library for classic as well as state-of-the-art computer vision and machine learning algorithms. In combination with Python Anaconda, you will have access to all the open-source computing libraries you could possibly ask for.Machine learning for OpenCV begins by introducing you to the essential concepts of statistical learning, such as classification and regression. Once all the basics are covered, you will start exploring various algorithms such as decision trees, support vector machines, and Bayesian networks, and learn how to combine them with other OpenCV functionality. As the book progresses, so will your machine learning skills, until you are ready to take on todays hottest topic in the field: Deep Learning.By the end of this book, you will be ready to take on your own machine learning problems, either by building on the existing source code or developing your own algorithm from scratch!What you will learnExplore and make effective use of OpenCVs machine learning moduleLearn deep learning for computer vision with PythonMaster linear regression and regularization techniquesClassify objects such as flower species, handwritten digits, and pedestriansExplore the effective use of support vector machines, boosted decision trees, and random forestsGet acquainted with neural networks and Deep Learning to address real-world problemsDiscover hidden structures in your data using k-means clusteringGet to grips with data pre-processing and feature engineeringAbout the AuthorMichael Beyeler is a Postdoctoral Fellow in Neuroengineering and Data Science at the University of Washington, where he is working on computational models of bionic vision in order to improve the perceptual experience of blind patients implanted with a retinal prosthesis (bionic eye). His work lies at the intersection of neuroscience, computer engineering, computer vision, and machine learning. Michael is an active contributor to several open-source software projects, and has professional programming experience in Python, C/C++, CUDA, MATLAB, and Android. Michael received a Ph.D. in Computer Science from the University of California, Irvine as well as a M.Sc. in Biomedical Engineering and a B.Sc. in Electrical Engineering from ETH Zurich, Switzerland. When he is not nerding out on brains, he can be found on top of a snowy mountain, in front of a live band, or behind the piano.Table of ContentsA Taste of Machine LearningWorking with Data in OpenCV and PythonFirst Steps in Supervised LearningRepresenting Data and Engineering FeaturesUsing Decision Trees to Make a Medical DiagnosisDetecting Pedestrians with Support Vector MachinesImplementing a Spam Filter with Bayesian LearningDiscovering Hidden Structures with Unsupervised LearningUsing Deep Learning to Classify Handwritten DigitsCombining Different Algorithms Into an EnsembleSelecting the Right Model with Hyperparameter TuningWrapping Up
Reviews
Be the first to review this e-book.
Write your review
Wanna review this e-book? Please Sign in to start your review.